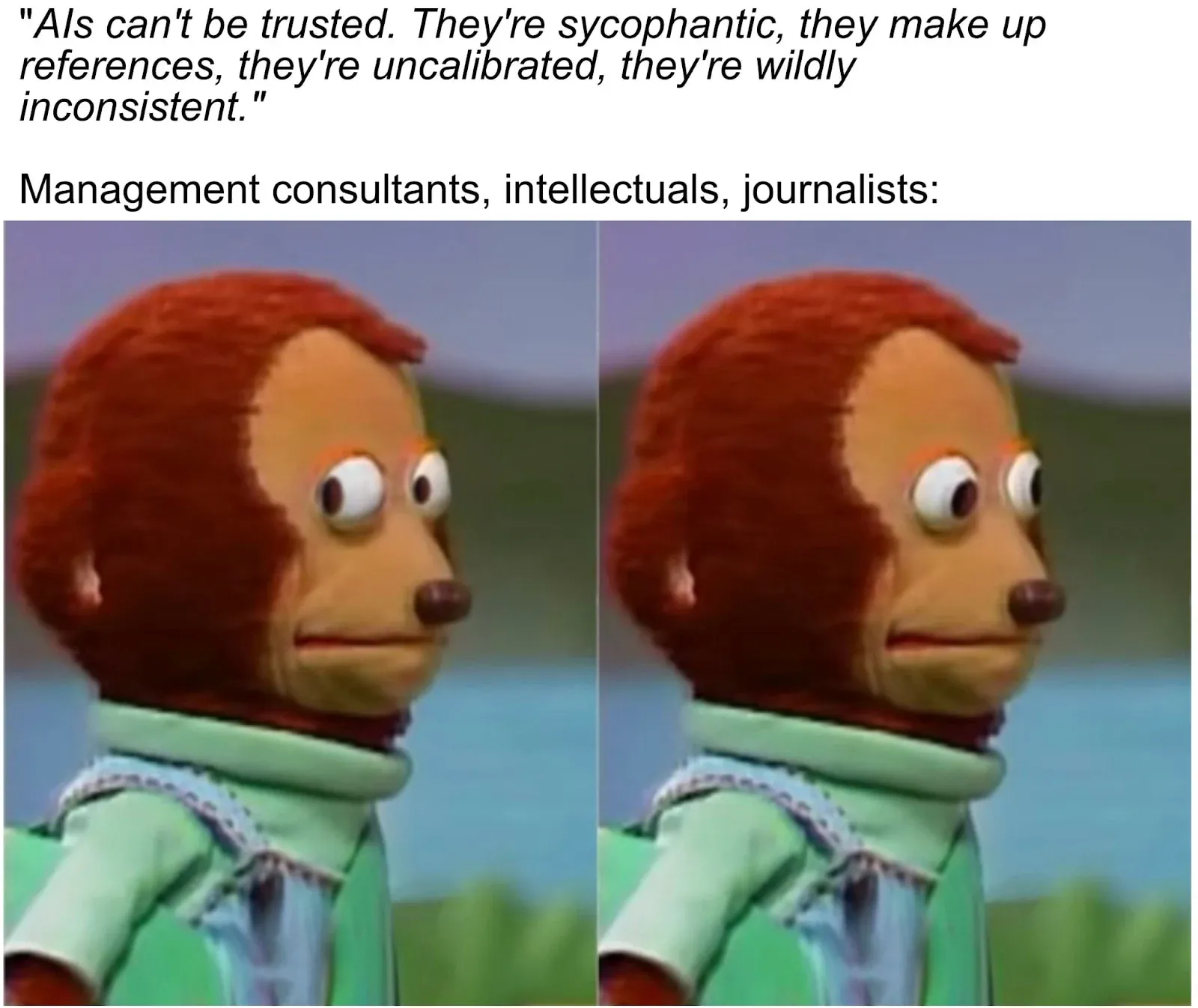
6 (Potential) Misconceptions about AI Intellectuals
Update
I recently posted this to the EA Forum, LessWrong, and my Facebook page, each of which has some comments.
Epistemic Status
A collection of thoughts I've had over the last few years, lightly edited using Claude. I think we're at the point in this discussion where we need to get the basic shape of the conversation right. Later we can bring out more hard data.
Summary
While artificial intelligence has made impressive strides in specialized domains like coding, art, and medicine, I think its potential to automate high-level strategic thinking has been surprisingly underrated. I argue that developing "AI Intellectuals" - software systems capable of sophisticated strategic analysis and judgment - represents a significant opportunity that's currently being overlooked, both by the EA/rationality communities and by the public. More fundamentally, I believe that a lot of people thinking about this area seem to have substantial misconceptions about it, so here I try to address those.
Background
Core Thesis
I believe we can develop capable "AI Intellectuals" using existing AI technologies through targeted interventions. This opportunity appears to be:
- Neglected: Few groups are actively pursuing this direction
- Important: Better strategic thinking could significantly impact many domains, including AI safety
- Tractable: Current AI capabilities make this achievable
- Relatively Safe: Development doesn’t require any particularly scary advances
Different skeptics have raised varying concerns about this thesis, which I'll address throughout this piece. I welcome deeper discussion on specific points that readers find particularly interesting or contentious.
The Current Landscape
Recent advances in large language models have dramatically raised expectations about AI capabilities. Yet discussions about AI's impact tend to focus on specific professional domains while overlooking its potential to enhance or surpass human performance in big-picture strategic thinking.
Consider these types of questions that AI systems might help address:
- What strategic missteps is Microsoft making in terms of maximizing market value?
- What metrics could better evaluate the competence of business and political leaders?
- Which public companies would be best off by firing their CEOs?
- Which governmental systems most effectively drive economic development?
- How can we more precisely assess AI's societal impact and safety implications? Are current institutional investors undervaluing these factors?
- How should resources be allocated across different domains to maximize positive impact?
- What are the top 30 recommended health interventions for most people?
We should seek to develop systems that can not only analyze these questions with reliability and precision, but also earn deep trust from humans through consistent, verifiable performance. The goal is for their insights to command respect comparable to or exceeding that given to the most credible human experts - not through authority or charisma, but through demonstrated excellence in judgment and reasoning.
Defining AI Intellectuals
An AI Intellectual would be a software system that can:
- Conduct high-level strategic analysis
- Make complex judgments
- Provide insights comparable to human intellectuals
- Engage in sophisticated research and decision-making
This type of intellectual work currently spans multiple professions, including:
- Business executives and management consultants
- Investment strategists and hedge fund managers
- Think tank researchers and policy analysts
- Professional evaluators and critics
- Political strategists and advisors
- Nonfiction authors and academics
- Public intellectuals and thought leaders
An AI intellectual should generally be good at answering questions like those posted above.
While future AI systems may not precisely mirror human intellectual roles - they might be integrated into broader AI capabilities rather than existing as standalone "intellectual" systems - the concept of "AI Intellectuals" provides a useful framework for understanding this potential development.
Another note on terminology: While the term "intellectual" sometimes carries baggage or seems pretentious, I use it here simply to describe systems capable of sophisticated strategic thinking and analysis.
Related Work
There's been some recent discussion circling this topic recently, mostly using different terminology.
- Lukas Finnveden has written broadly on AI and Epistemics
- Chris Leong has written about "Wise AI Advisors"
- benwr recently posted about "Strategically Superhuman Agents"
- At QURI, we've been discussing a lot of related topics. One conception was "Advancing Wisdom and Intelligence."
- Owen Cotton-Barratt and others held a competition on the "Automation of Wisdom and Philosophy"
Here I use the phrase "AI Intellectuals" to highlight one kind of use, but I think that this fits neatly into the above cluster. I very much hope that over the next few years there's progress on these ideas and agreement on key terminology.
Potential Misconceptions
Misconception 1: “Making a trustworthy AI intellectual is incredibly hard”
Many people seem to hold this belief implicitly, but when pressed, few provide concrete arguments for why this should be true. I’ll challenge this assumption with several observations.
The Reality of Public Intellectual Work
The public intellectuals most trusted by our communities—like Scott Alexander, Gwern, Zvi Mowshowitz, and top forecasters—primarily rely on publicly available information and careful analysis, not privileged access or special relationships. Their key advantage is their analytical approach and thorough investigation of topics, something that’s easy to imagine AI systems replicating.
Evidence from Other AI Progress
We're already successfully automating numerous complex tasks—from large-scale software engineering to medical diagnosis and personalized education. It's unclear why high-level strategic thinking should be fundamentally more difficult to automate than these areas.
An Executive Strategy Myth
There's a common misconception that business executives possess superior strategic thinking abilities. However, most executives spend less than 20% of their time on high-level strategy, with the bulk of their work focused on operations, management, and execution. It seems likely that these leaders weren’t selected for incredibly great strategic insight - instead they excelled at a combination of a long list of skills. This would imply that many of the most important people might be fairly easy to both help or to outperform with strategy and intellectual work specifically.
My Experience at QURI
Between Guesstimate and QURI, I’ve spent several years making decision analysis tools, and more recently Squiggle AI to automate this sort of work. I’ve been impressed by how LLMs can be combined with some basic prompt engineering and software tooling to generate much better results.
AI forecasting has been proven to be surprisingly competent in some experiments so far. Online research is also starting to be successfully automated, for example with Elicit, Perplexity, and recently Deep Research. I’ve attempted to map out many of the other tasks I assume might be required to do a good job, and all seem very doable insofar as software projects go.
Overall I think the technical challenges look very much doable for the right software ventures.
General Epistemic Overconfidence
I’ve noticed a widespread tendency for people to overestimate their own epistemic abilities while undervaluing insights from more systematic researchers. This bias likely extends to how people evaluate AI's potential for strategic thinking. People who are overconfident in their own epistemics are likely to dismiss other approaches, especially when those approaches come to different conclusions. While this might mean that AI intellectuals would have trouble being listened to, it also could imply that AIs could do a better job at epistemic work than many people would think.
Related Discussion around AI Alignment
Some AI safety researchers argue that achieving reliable AI epistemics will be extremely challenging and could present alignment risks. The Ontology Crisis discussion touches on this, but I haven't found these arguments particularly compelling.
There seems to be a decent segment of doomers who expect that TAI will never be as epistemically capable as humans due to profound technical difficulties. But the technical difficulty in question seems to vary greatly between people.
Strategy and Verifiability
A common argument suggests that "AIs are only getting good at tasks that are highly verifiable, like coding and math." This implies that AI systems might struggle with strategic thinking, which is harder to verify. However, I think this argument overlooks two main points.
First, coding itself demonstrates that AIs can be strong at tasks even when perfect verification isn't possible. While narrow algorithm challenges are straightforward, AI systems have shown proficiency in (and are improving at) harder-to-verify aspects like:
- Code simplicity and elegance
- Documentation quality
- Coherent integration with larger codebases
- Maintainability and scalability
Second, while high-level strategy work can be harder to verify than mathematical proofs, there are several meaningful ways to assess strategic capabilities:
Strong verification methods:
- Having AI systems predict how specific experts would respond to strategic questions after deliberation
- Evaluating performance in strategy-heavy environments like Civilization 5
- Assessing forecasting ability across both concrete and speculative domains
Lighter verification approaches:
- Testing arguments for internal consistency and factual accuracy
- Comparing outputs between different model sizes and computational budgets
- Validating underlying mathematical models through rigorous checks
- Requiring detailed justification for unexpected claims
It's worth noting that human intellectuals face similar verification challenges, particularly with speculative questions that lack immediate feedback. While humans arguably are better right now at hard-to-verify tasks, they still have a lot of weaknesses here, and it seems very possible to outperform them using the strategies mentioned above.
Strategic Analysis and EA
While EA and AI Safety strategy may be more sophisticated than outside perspectives, this might represent a relatively low bar. I can envision near-term AI systems providing more trustworthy analysis on these topics than the current researchers we have now.
Misconception 2: “There's some unreplicable human secret sauce”
A common argument against AI intellectuals is that humans possess some fundamental, unreplicable, quality essential for high-level thinking. This "secret sauce" argument takes many forms, but all suggest there's some critical human capability that AI systems can never achieve (at least, before TAI).
Some examples of “secret sauce” arguments I’ve heard:
- "AIs can’t handle deep uncertainty."
- “AIs can only answer questions. They can’t come up with them.”
- "AI can’t generate truly original ideas like humans can."
- “AIs can’t generalize moral claims like humans can.”
- “AIs can’t understand human values as well as individual humans do.”
- “AIs always optimize for proxy metrics, in ways that humans don’t.”
My guess is that most of these aren’t that serious and don’t represent cruxes for their proponents, mainly because I haven’t seen most of them be rigorously argued for.
Due to the number of examples it’s hard for me to argue against all, but I’m happy to go more into detail on any specific ones if asked.
I can say that none of the examples seem clearly correct to me. It’s possible that one will be a limiting factor, but this would surprise me, and I’d be willing to bet on Manifold against.
Misconception 3: “AI Intellectuals will follow an all-or-nothing trajectory”
The Mistaken View
There's a prevalent belief that AI intellectual capabilities will follow a binary trajectory: either completely useless or suddenly revolutionary. This view is particularly common in discussions about AI and forecasting, where some argue that AI systems will be worthless until they surpass human performance, at which point human input becomes obsolete.
I think that having this view would lead to other important mistakes about the viability of AI Intellectuals, so I’ll address it in some detail.
The Reality of Technological Progress
This binary thinking misunderstands how technology typically develops:
- Most technologies advance gradually along an S-curve
- Early versions provide modest but real value
- Improvements compound over time
- Integration happens incrementally, not suddenly
A Better Model: The Self-Driving Car Analogy
Consider how self-driving technology has evolved:
- Started with basic driver assistance (Level 1)
- Gradually added more sophisticated features
- Each level brought concrete benefits
- Full autonomy (Level 5) represents the end of a long progression
How I Think AI Intellectuals Will Likely Develop
We're already seeing this pattern with AI intellectual tools:
- Initial systems assist with basic research, ideation, and fact-checking
- Capabilities expand to draft generation and analysis
- Progress in trustworthiness and reliability happens gradually
- Trust increases, in rough accordance to trustworthiness (for example, with people gradually using LLMs and developing intuitions about when to trust them)
Even with rapid AI progress (say, TAI in 5 years), this evolution would likely still take considerable time (perhaps 3 years, or 60% of TAI time left) to achieve both technical capability and institutional trust. But we can gradually scale it up, meaning it will be useful at each step and predictable.
I assume that early “full AI Intellectuals” will be mediocre but still find ways to be useful. Over time these systems will improve, people will better understand how much they can trust them, and people will better learn where and how to best use them.
Misconception 4: “Making a trustworthy AI intellectual is incredibly powerful/transformative”
I’ve come across multiple people who assume that once AI can outperform humans at high-level strategic questions, this will almost instantly unlock massive amounts of value. It might be therefore assumed that tech companies would be highly incentivized to do a great job here, and that this area won’t be neglected. Or it might be imagined that these AIs will immediately lead to TAI.
I think those who have specialized in the field of forecasting often recognize just how low in importance it often is. This doesn’t mean that forecasting (and similarly, intellectual work) is not at all useful - but it does mean that we shouldn’t expect much of the world to respond quickly to improvements in epistemic capabilities.
Few people care about high-quality intellectual work
The most popular intellectuals (as in, people who provide takes on intellectuals that others listen to) are arguably much better at marketing than they are at research and analysis. In my extended circles, the most popular voices include Malcolm Gladwell, Elon Musk, Tim Ferris, Sam Harris, etc.
Arguably, intellectual work that’s highly accurate has a very small and often negative mimetic advantage. Popular figures can instead focus on appearing confident and focus on other popularity measures, for instance.
Tetlock’s work has demonstrated that most intellectual claims are suspect, and that we can have forecasting systems that can do much better. And yet, these systems are still very niche. I’d expect that if we could make them 50% more accurate, perhaps with AIs, it would take time for many people to notice. Not many people seem to be paying much attention.
High-quality intellectual work is useful in a narrow set of areas
I think that intellectual work often seems more informative than it is actually useful.
Consider:
- Often, conventional wisdom is pretty decent. There aren’t often many opportunities for much better decisions.
- In cases where major decisions are made poorly, it’s often more out of issues like conflicts of interest or ideological stubbornness than it is intelligence. There’s often already some ignored voices recommending the correct moves - adding more voices wouldn’t exactly help.
- Getting the “big picture strategy” right is only one narrow piece of many organizations. Often organizations do well because they have fundamental advantages like monopoly status, or because they execute well on a large list of details. So if you can improve the “big picture strategy” by 20%, that might only lead to a 0.5% growth in profits.
Forecasting organizations have recently struggled to produce decision-relevant estimates. And in cases where these estimates are decision-relevant, they often get ignored. Similar to intellectuals. This is one major reason why there’s so little actual money in public forecasting markets or intellectual work now.
All that said, I think that having better AI intellectuals can be very useful. However, I imagine that they can be very gradually rolled out and that it could take a long time for them to be trusted by much of the public.
There are some communities that would be likely to appreciate better epistemics early on. I suspect that the rationality / effective altruism communities will be early here, as has been the case for prediction markets, bayesianism, and other ideas.
Misconception 5: “Making a trustworthy AI intellectual is inherently dangerous”
Currently, many humans defer to certain intellectuals for their high-level strategic views. These intellectuals, while influential, often have significant limitations and biases. I believe that AI systems will soon be able to outperform them on both capabilities and benevolence benchmarks, including measures like honesty and transparency.
I find it plausible that we could develop AI systems that are roughly twice as effective (in doing intellectual work) as top human intellectuals while simultaneously being safer to take advice from.
If we had access to these "2x AI intellectuals," we would likely be in a strictly better position than we are now. We could transition from deferring to human intellectuals to relying on these more capable and potentially safer AI systems. If there were dangers in future AI developments, these enhanced AI intellectuals should be at least as competent as human experts at identifying and analyzing such risks.
Some might argue that having 2x AI intellectuals would necessarily coincide with an immediate technological takeoff, but this seems unlikely. Even with such systems available today, I expect many people would take considerable time to develop trust in them. Their impact would likely be gradual and bounded - for instance, while they might excel at prioritizing AI safety research directions, they wouldn't necessarily be capable of implementing the complex technical work required.
Of course, there remains a risk that some organizations might develop extremely powerful AI systems with severe epistemic limitations and potentially dangerous consequences. However, this is a distinct concern from the development of trustworthy AI intellectuals.
It’s possible that while “2x AI intellectuals” will be relatively safe, “100x AI intellectuals” might not be, especially if we reached it very quickly without adequate safety measures. I would strongly advise for gradual ramp-up. Start with the “2x AI intellectual”, then use it to help us decide on next steps. Again, if we were fairly confident that this “2x AI intellectual” were strictly more reliable than our existing human alternatives, then this should be strictly better at guiding us in the next steps.
Lastly, I might flag that we might not really have a choice here. If we radically change our world using any aspects of AI, we might require much better intellectual capacity in order to not have things go off the rails. AI intellectuals might be one of our best defenses against a dangerous world.
Misconception 6: “Delegating to AI means losing control”
This concern often seems more semantic than substantive. Consider how we currently interact with technology: few people spend time worrying about the electrical engineering details of their computers, despite these being crucial to their operation. Instead, we trust and rely upon expert electrical engineers for such matters.
Has this delegation meant a loss of control? In some technical sense, perhaps. But this form of delegation has been overwhelmingly positive - we've simply entrusted important tasks to systems and experts who handle them competently. The key question isn't whether we've delegated control, but whether that delegation has served our interests.
As AI systems become more capable at making and explaining strategic choices, these decisions will likely become increasingly straightforward and convergent. Just as we rarely debate the mechanical choices behind our refrigerators' operation, future generations might spend little time questioning the implementation details of governance systems. These details will seem increasingly straightforward, over-determined, and boring.
Rather than seeing this as problematic, we might consider it liberating. People could redirect their attention to whatever areas they choose, rather than grappling with complex strategic decisions out of necessity.
While I don’t like the specific phrase “lose control”, I do think that there are some related questions that are both concrete and important. For example: "When humans delegate strategic questions to AIs, will they do so in ways that benefit or harm them? Will this change depending on the circumstance?" This deserves careful analysis of concrete failure modes like overconfidence in delegation or potential scheming, rather than broad concerns about "loss of control."
Further Misconceptions and Beyond
Beyond the six key misconceptions addressed above, I've encountered many other questionable beliefs about AI intellectuals. These span domains including:
- Beliefs about what makes intellectual work valuable or trustworthy
- Claims about what strategic thinking fundamentally requires
- Arguments about human vs. AI epistemics
- Questions about institutional adoption and integration
While I could address each of these, I suspect many aren't actually cruxes for most skeptics. I've noticed a pattern where surface-level objections often mask deeper reservations or simple lack of interest in the topic.
This connects to a broader observation: there's surprisingly little engagement with the concept of "AI intellectuals" or "AI wisdom" in current AI discussions. Even in communities focused on AI capabilities and safety, these topics rarely receive sustained attention.
My current hypothesis is that the specific objections people raise often aren't their true bottlenecks. The lack of interest might stem from more fundamental beliefs or intuitions that aren't being explicitly articulated.
Given this, I'd particularly welcome comments from skeptics about their core reservations. What makes you uncertain about or uninterested in AI intellectuals? What would change your mind? I suspect these discussions might represent the most productive next steps.
Thanks to Girish Sastry and Vyacheslav Matyuhin for feedback on this post.