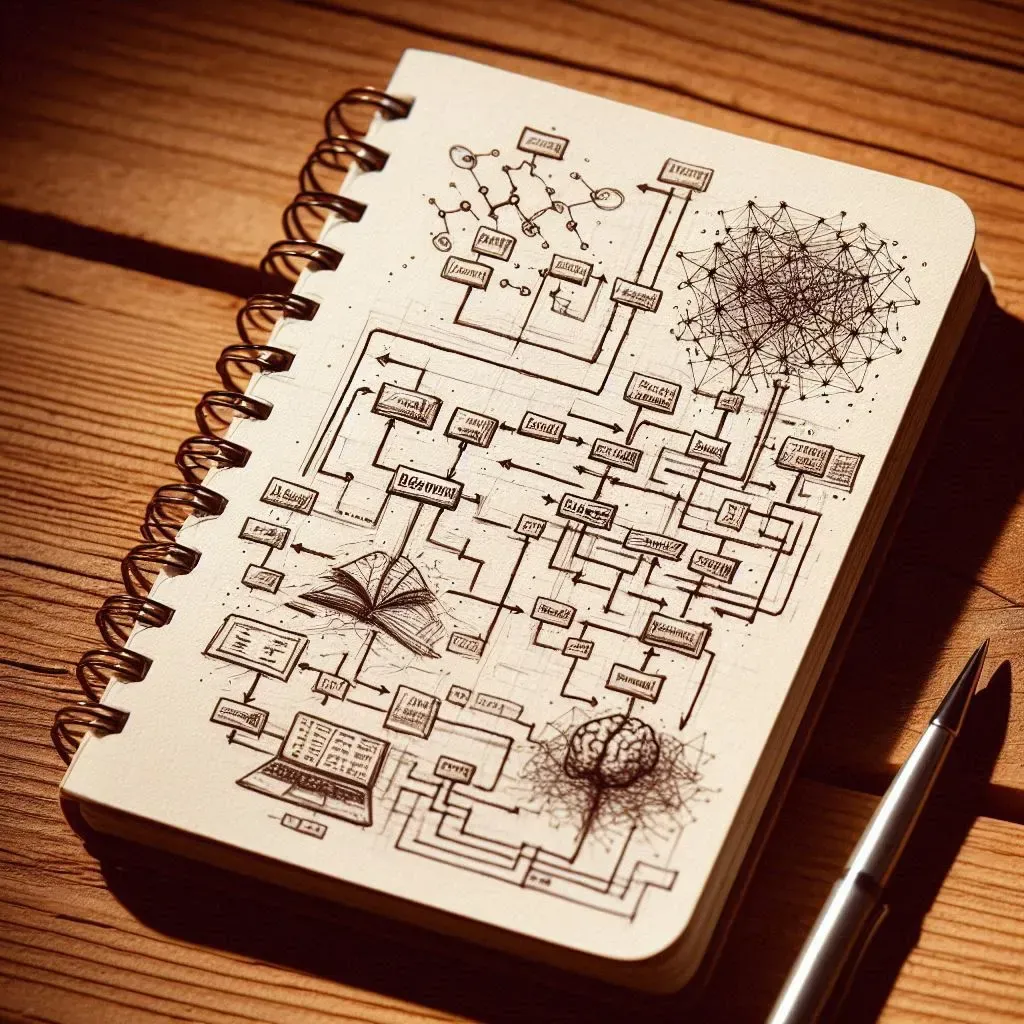
Current Claims and Cruxes on LLM Forecasting & Epistemics
I think that recent improvements in LLMs have brought us to the point where LLM epistemic systems are starting to be useful. After spending some time thinking about it, I've realized that such systems, broadly, seem very promising to me as an effective altruist intervention area. However, I think that our community has yet to do a solid job outlining what this area could look like or figuring out key uncertainties.
This document presents a rough brainstorm on these topics. While I could dedicate months to refining these ideas, I've chosen to share these preliminary notes now to spark discussion. If you find the style too terse, feel free to use an LLM to rewrite it in a format you prefer.
I believe my vision for this area is more ambitious and far-reaching (i.e. not narrow to a certain kind of forecasting) than what I've observed in other discussions. I'm particularly excited about AI-heavy epistemic improvements, which I believe have greater potential than traditional forecasting innovations.
I'm trying to figure out what to make of this regarding our future plans at QURI, and I recommend that other organizations in the space consider similar updates.
Key Definitions:
- Epistemic process: A set of procedures to do analysis work, often about topics with a lot of uncertainty. This could be “have one journalist do everything themselves”, to a complex (but repeatable) ecosystem of multiple humans and software systems.
- LLM-based Epistemic Process (LEP): A system that relies on LLMs to carry out most or all of an epistemic process. This might start at ~10% LLM-labor, but can gradually ramp up. I imagine that such a process is likely to feature some kinds of estimates or forecasts.
- Scaffolding: Software used around an LLM system, often to make it valuable for specific use-cases. In the case of an LEP, a lot of scaffolding might be needed.
1. High-Level Benefits & Uses
Claim 1: If humans could forecast much better, these humans should make few foreseeable mistakes. This covers many mistakes, particularly ones we might be worried about now.
- Someone deciding about talking to a chatbot that can be predicted to be net-negative (perhaps it would create an unhealthy relationship) could see this forecast and simply decide not to start the chat.
- Say that a person’s epistemic state could follow one of four trajectories, depending on some set of reading materials. For example, one set is conspiratorial, one is religious, etc. Good forecasting could help forecast this and inform the person ahead of time.
- Note that this can be radical and perhaps dangerous. For example, a religious family knowing how to keep their children religious with a great deal of certainty.
- Say that one of two political candidates is predictably terrible. This could be made clear to voters who trust said prediction.
- If an AI actor is doing something likely to be monopolistic or dangerous, this would be made more obvious to itself and those around it.
- Note: There will also be unforeseeable mistakes, but any actions that we could do that are foreseeably-high-value for them, could be predicted. For example, general-purpose risk mitigation measures.
Claim 2: Highly intelligent / epistemically capable organizations are likely to be better at coordination.
- This might well mean fewer wars and conflict, along with corresponding military spending.
- If highly capable actors were in a prisoner's dilemma, the results could be ugly. But very often, there’s a lot of potential and value in not getting into one in the first place.
- Evidence: From The Better Angels of Our Nature, there’s significant evidence that humanity has become significantly less violent over time. One potential exception is that large risks of conflict between large actors might have increased, but these last bits are some of the most difficult to coordinate, so this might not be too surprising.
Claim 3: The harm caused by altruistic development of epistemic improvements could be capped by a self-enforcing loop.
- Agents developing epistemics should use the tools they build to decide on the net value of further improvements. This is a very important variable to get right.
- If it ever seems like future work will be negative in expected value, progress could and should be stopped.
Claim 4: Better forecasting would lead to more experimentation and innovation (“weirdness”).
- Radical technology projects are well-known to be high-risk. High returns when they go well, high losses if they don’t. This means that there’s a high value of information in being more correct about outcomes early on.
- If people could become much better at forecasting, then it’s expected that they will become better at picking innovations to try. Bad projects would be avoided more often, and the expected value of innovation would increase.
Claim 5: AI forecasting/epistemics will be much more deeply integrated into the world than current prediction markets.
- First, note that current prediction markets / tournaments are highly niche and overlooked. So AI systems would have to be more accepted, to be very valuable.
- Unlike current “human forecasters”, AI forecasting can be embedded deeply into other software. There would likely be better AI forecasting for repeated decisions, like devops procedures, edge cases in insurance, AI agents, etc.
- If “AI forecasters” were really solid and cheap, we’d expect them to improve many complex software workflows. Existing “human forecasters” are hard to directly make much money from, but these AI systems would be much easier to do so.
- They would likely be integrated in many other systems, such as:
- Overseeing product sales chances
- Estimating changes in accounting, from one quarter to the next
- Estimating project lengths and budgets
- Deciding the best LLM to use for a certain AI workflow, or the best workflow to follow.
- Note that many developments in "AI epistemics" are just regular enhancements to LLMs. There's no clear line between "AI forecasting tech" and "improvements in LLMs that make them better general-purpose reasoners."
Claim 6: Even "straightforward" AI forecasting systems should look very different from human judgemental forecasting systems
- I've found others seem to imagine AI forecasting systems as simply replacing humans in prediction tournaments and prediction markets. I think think this is perhaps a decent first model, but not at all how things would end up.
- Prediction markets / tournaments are very much optimized for competitive humans, in cases where humans need to be incentivized. AI systems have very different constraints, so will need very different systems.
- Formal resolutions won't be necessary to incentivize AIs, after they are trained.
- Forecasting can be done on-demand, as the AI is always available. So questions and forecasts might not have to be done continuously, before being read by end-users.
- There's unlikely to be a need to have many AIs compete against each other, like in a traditional tournament, in most cases.
- Modern prediction platforms don't use advanced inputs like scorable functions. Arguably these would be too complex for many humans, but this is unlikely to be the case for AIs.
2. Failure Modes
- Nonprofit efforts in the space are not effective
- Claim: The most likely failure mode for projects in this area is that they will fail to make an impact.
- If LLM abilities dramatically outpace agent / scaffolding architectures, then the only useful work here might be done by large LLM labs.
- There will likely be commercial entities taking care of the most obvious and low-hanging market opportunities. It’s not clear how to expect this area to change over time. It's possible that nonprofit / EA efforts here would have trouble finding a strong fit.
- Claim: The most likely failure mode for projects in this area is that they will fail to make an impact.
- Forecasting could be used for net-bad activities
- AI Acceleration
It’s possible that epistemic improvements would be used by AI capabilities actors to increase capabilities and not make other informed choices.
Claim: I think this is unlikely to be the net outcome. This would require these organizations to become smarter about making AIs, but not much smarter about making them safe, including for their own benefit. It would also require the other groups that could block them to become smarter - some of these groups include other non-LLM companies and other highly motivated actors who wouldn't want AI to go poorly.
One crux here is if the AI developers will both be much better at integrating AI epistemic improvements in their internal workflows, and if these systems won't convince or help them with AI alignment. This could be a really bad outcome, so it's important to continue to pay attention to. - Malicious Governments
Governments like China or North Korea could use better epistemic systems to better control their populations. My guess is that great epistemic systems will likely do some damage here. I think that overall the improvements (like improving western governments) will more than outweigh that, but this is something to pay attention to.
Overall, I don’t see reasons to think that better epistemics will dramatically change existing power balances in ways that are yet predictable. I think this is true for most technologies I could imagine, even many of the most beneficial ones. - Malicious Corporations
There are some situations where companies could be far better at forecasting than consumers would be, leading to a large power imbalance. If companies have much more information about customers than users have about themselves or these companies, that could lead to them being taken advantage of. It seems important to use governments (hopefully with better epistemic infrastructure) to take active steps against this. It seems important here to work to make sure that epistemic advances get distributed equitably.
- AI Acceleration
- Intelligent systems lead to more complexity, leading to a less stable world
Arguably as humans become more intelligent, human civilization becomes more complex. Complexity has a cost, and at some points, these costs can become detrimental.
As you might expect, I think this is a predictable disaster, meaning that better prediction abilities would help here. I’d expect agents to be able to make decisions about one step at a time, and for each, see if the benefits are worth the costs. In addition, forecasting will make many things simpler and more handleable. See sources like Thinking Like a State to understand ways that power structures actively force systems to become simpler - sometimes even too much so. - There's too much confusion over the terminology and boundaries, so research is stunted
"Epistemics" is a poorly understood term. "Judgemental Forecasting" is still very niche. The term "forecasting" is arguably too generic. It seems very possible that this area will struggle in finding agreed-upon and popular framings and terminology, which could contribute to it be neglected. I think more terminological and clarification work here could be valuable. This is similar to the idea of Distillation and Research Debt.
3. Viability
Claim 1: All/Almost all parts of a conventional epistemic process can be automated with LLMs.
LLMs can:
- Generate forecasting questions
- Resolve forecasting questions
- Gather data from computers
- Write specific questions to expert - a la interviews
- Make forecasts
- Make visualizations and dashboards of forecasts
- Personalize information for specific people
Note: “Can be automated” doesn’t mean “easy to automate in a cost-effective or high-quality way.”
Claim 2: A lot of communication is low-entropy, especially with knowledge of the author.
- Low-entropy means “predictable by a decent AI agent, knowing information about the author’s history”.
- Evidence: We currently have systems that predict what people will say in very limited extents - i.e. for email. These typically don’t have much information about the user. I expect this sort of thing will become more widely integrated and capable.
- Evidence: When I write, I feel like much of my communication is highly predictable. I’m able to use Claude to do a lot for me. It’s not there yet - but the setup now is incredibly basic (it doesn’t even have my historic writing), and the tech is improving.
- At some point, we could make decent approximations of what many people believe on many topics. This could help us greatly speed up communication - AI systems would just need the pieces they can’t predict.
Claim 3: LLM-epistemics should be easier to study and optimize than human-epistemics.
They can be:
- Reproducible (models don’t change over time)
- Versioned (if updates are made to an LLM, this new LLM can be separately studied)
- Pure (similar inputs, with seeds, produce the same outputs)
- Scalable (much easier for 10 researchers to study one LLM in parallel, than studying one human. Can also reuse the same exact LLM in many places, or researchers could run 500 LLM runs in parallel.)
- Investigatable (People have privacy concerns. LLMs don’t, so we don’t need to be cautious about making them feel bad with public evaluations)
- Modifiable (We can’t freely change parts of most people’s knowledge or abilities, but we can with LLMs.
- We can experiment with a large number of isolated changes.
- We can’t wipe a person’s memory in order to backtest a human-based epistemic process, but we (partially) do this for LLMs, by providing them access to specific data sets.
- Related point: One challenge to note though is that the training data is particularly optimized for immediate times after the LLM was trained. We should probably only test LLMs in forecasting scenarios after they have been trained, and assume that their quality will degrade for forecasts they make far out from their training time. That said, this still is overall far more standardizable than with humans, which are typically our main alternative.
Add to that, that LLMs are often cheaper and better than many humans, and overall, these systems are hard to ignore.
Evidence:
- LLM R&D has dramatically risen recently and has resulted in major gains (>$2T) to company valuations. Human R&D around epistemics and reasoning has had nothing like this recently.
- We have far more studies and data about LLM performance than we do about important scientists, intellectuals, or decision-makers. There are of course some human studies (a la the Good Judgement Project), but these are expensive and thus few and very narrow.
Subclaim: Even if we just wanted to understand ideal human epistemics better, one reasonable method might be to make strong LLM systems and study them, because these are much easier to study, and will likely lead to some generalizable findings.
Claim 4: LLM epistemic integration will be gradual.
Practically speaking LLMs and Humans will work together side-by-side in epistemic processes for some time. I expect LLMs-epistemics to follow a similar trajectory to self-driving. First, it starts with light augmentation. Then it moves to “fully automated in most use cases, but occasionally needs oversight.” As with autonomous driving, Level 5 is very difficult.
- Subpoint: Don’t ask “When will LLMs be better than humans at [forecasting|writing|research],” Ask questions more like, “what percentage of work done in [forecasting|writing|research] be automated”, or, “what ratio of Manifold Karma will be won by bots vs. humans vs. hybrids, over time?” I’d expect to see gradual gains here.
Subclaim: I expect that LLM-based forecasters will gradually dominate open platforms like Manifold and Metaculus. As this happens, it might scare off many of the human forecasters. If this is not handled gracefully, it could lead to these platforms feeling like ghost towns and becoming irrelevant, in favor of people using more direct AI systems.
4. Scaffolding
Previous LLM-based forecasting systems have included some amounts of scaffolding.
Claim 1: LLM-based epistemic systems will likely require a lot of scaffolding, especially as they get ambitious.
I.E. software, databases, custom web applications, custom batch job pipelines, etc.
Claim 2: Scaffolding-heavy systems have significant downsides.
- Difficult to study. Unlike single LLMs, scaffolded systems can feature:
- High costs of deployment.
- No/little ability to roll-back.
- Frequent changes.
- Internal substructures that are highly variable between different implementations.
- External-facing APIs that are very different between different implementations.
- Evidence: The previously mentioned LLM-based systems have some of these failures - and these are simple systems compared to what we might have later.
- Subclaim: If scaffolding is to be expected / needed, it could be important to plan for research measures that take this into account. This will require specialized attention.
- Subclaim: It seems important for there to be some “portable/packaged” LEPs, that could easily be shared, versioned, deployed, etc. This would be useful for research and benchmarks/baselines.
Claim 3: Scaffolding-heavy systems are likely to be centralized.
- In general, complex web projects are centralized.
- This means that scaffolding-heavy setups will favor startups and in-house teams.
- This means that it's easy for many of these systems to be useless. Users might only interact with 1-2 systems, so any smaller competitors might get totally ignored, even if they have a few unusually great features.
- It’s possible that there could be some clean lines of separation between different components. Some lines seem simple to try out, but experimentation and thinking is needed to develop a better idea. Thinking/planning here seems good.
- The more it’s split up into understood components, the easier it will be to research, optimize, and proliferate each part.
Claim 4: Scaffolding-heavy systems are expensive, and thus difficult to incentivize people to build.
- Most forecasting competitions now pay out fairly small amounts. This makes it difficult to incentivize scaffolding.
- I don’t expect people to independently create great scaffolding in order to win <$50k.
- My impression is that very little forecasting on Metaculus and Manifold is automated with LLMs. (There is one recent Metaculus competition here, but this is an isolated and forced use.)
- It’s also difficult to share and maintain scaffolding. You need to make custom web UIs, you might have to set up billing infrastructure for payments, etc. In a very large forecasting ecosystem with a lot of money, forecasters would subscribe to scaffolding systems, in the same way the professional traders subscribe to trading software and services.
- This means that we might well see slower progress here / less diversity.
Claim 5: New technologies or startups could greatly change and improve scaffolding.
Right now there are some startups and open-source frameworks to help with general-purpose scaffolding, but these are known to be lacking. It's possible that much better systems will be developed in the future, especially because there's a lot of money in the space.
If this happens, that could hypothetically make powerful LLM epistemic tools dramatically easier to create.
One annoying challenge is that change in the field could lessen the importance of early experimentation. It could be the case that early projects focus heavily on some specific scaffolding frameworks, only for other frameworks to become more powerful later.
A possible successful world could be one where different teams make "GPTs" that can integrate nicely with other workflows and interact with each other. Ideally these wouldn't be restricted to any one platform.
Crux: Scaffolding vs. Direct LLM Improvements
One risk around scaffolding is that a lot of key functionality that scaffolding would be used for might be implemented directly in the inference of LLMs. For example, long-term planning could be done simply with scaffolding, but AI developers are also iterating on implementing it directly into the LLM layer.
Another example is that of editing text. If you have a long text document and want to use an LLM to make a few edits, it would currently attempt to rewrite the entire document, at a fairly slow speed. This could be improved with scaffolding by instead asking it to write a git diff and then applying that using traditional code. Or it could be solved on the inference layer directly, as discussed here.
If it is the case that most future improvements are done directly with the LLMs, then that could significantly change what work will be valuable. For example, if there are only a few leading LLM developers, then it might ultimately be up to them to actually add any functionality.
5. Subcomponents
As stated above, it’s hard to determine the best subdivisions of LEPs / LLM-Epistemic Scaffolding.
Here’s one attempt, to provide a simple picture.
1. Data-collection
- Many LLM services are already coming out that can help quickly put data, including private data, together, for different needs. See Apple Intelligence as one service that would work for people’s personal data.
- There are hundreds of startups in the data-collecting and data-management space. This problem can easily be split up more.
- Example performance question:
“Provided with resources R, gather as much data as you can, from this list of desired data, about X.”
The results of this will likely be obvious (it’s likely to be clear what data is accessible), so scientific testing might not be needed.
2. World Model
- “World model” here likely means, “A set of stored symbolic and probabilistic assumptions about the world / people / organizations.”
- World models will likely include software programs and writing, instead of only relying on existing LLMs.
- I expect that there could be workflows to LEPs to contribute to world models that will later give it better forecasting / reasoning abilities. The forecasting components could build the world models.
- Existing online data has very minimal attempts at providing accessible world models. These would ideally be probabilistic, immutable, shareable, collaborative, etc.
- Tools like Squiggle Hub are meant to be used here. Formal ontologies and knowledge graphs are likely to be useful.
- Some world models could be directly interesting to humans, for the presentation layer. Others might primarily be used by LLMs. For these, the world model is a form of a cache / collaboration.
- It seems possible/likely that world models won’t be unique to LEPs. Instead, many separate LEPs can use the same world models.
- World models could contain (or complete be) text descriptions of outcomes. For example, 10 compressed pages explaining how the next 10 years will go for a specific region.
- It’s not clear yet what the best representations are for LLMs to store and fetch data about its understanding of specific things.
- Example performance question:
“Given a forecasting LLM L, and forecasting question Q, contribute a world model that will result in L being highly accurate.”
3. Human Elicitation
- It’s often useful to just go out and directly ask questions to people, or give them surveys. Strong LEPs should ideally be able to design these procedures to maximize information value, then execute them autonomously.
- A system might realize that it would be useful to get a very specific expert to give their take on something. It could then email them, and perhaps offer a financial reward.
- A different system might generate a bunch of specific binary questions for a group of people it wants to elicit information from. Maybe it’s trying to estimate aspects of their preferences, and information would help.
- Example performance question:
“Given that you want to get as much useful new data about topic X, using existing research Y, use resources R to send off elicitions and gather data from humans.”
4. Data Prioritization
- The task here is, “Given the potential to fetch from data source D, and resources R, fetch the most useful data for providing marginal insight on unknown variables V.”
- Summarizing and displaying such work could also be useful. But doing so for humans would be very different to doing so for other LLMs.
- It seems like with LLMs that are capable of providing quick and accurate forecasts, we could use reinforcement learning and similar algorithms to do a good job here. For any set of data, we could quickly measure the improvement in accuracy using that data.
5. Numeric Modeling / Fermi Calculations
- Make great complex Python/Excel/Squiggle models/estimates.
- One reasonable approach to fermi estimation is “Try a bunch of very different approaches, then mix the answers together.”
- We’ve seen a lot of evidence that LLMs can generate working Python/Squiggle code. However, I don’t believe that there has been much optimization in this area yet.
- It seems easy to iterate on, and generate, great data of strong examples of this. That could be then used for LLM training / fine-tuning.
- Example performance question:
“Estimate variable V as well as you can, using up to N LLM calls, and no other calls.”
6. Decomposition / Amplification
There are many potential strategies to "break a problem down" and solve different parts with different threads of LLMs.
For example:
- Chain-of-Thought prompting
- Mixture of experts
- Ensemble Averaging
- Debate (i.e. Safety via Debate)
- Fact verification
Arguably, numeric modeling is a form of decomposition/amplification, I just separated it to draw attention to its uniqueness.
This is an active and popular area of ML research. Some of these techniques are incorporated in the LLM inference layer, but some are also used in scaffolding.
I'm sure there's a lot more work to do here. It's less clear where smaller nonprofit research can be most effective, but I suspect that there are some good options.
Many methods might be unique to forecasting or specific epistemic workflows. For example:
- Try to identify key cruxes or crucial considerations, and then investigate them
- Automated debate mapping
- Identify Scissor statements, in part to help personalize research
- Use discernment to clarify and decompose key terminology
- Breaking down forecasting questions into sub-questions, perhaps with bayesian networks.
Arguably, the rationality and effective altruist communities might be very well suited to work in this area. There's been a lot of writing on how to systematically break hard problems down, now people would need to experiment with encoding these systems into automated workflows.
7. Question Generation
- Come up with questions to be researched or forecasted on.
- This might well be done by other listed components - for example, it could simply combine "Idea Generation" with "Forecasting" to come up with potential questions, and guess which will be the most important and tractable. It also might be better done under the "Presentation" question. However, I suspect that there will be unique research and development for this problem, especially because it's a major part of existing forecasting systems.
- Example performance question:
“Write 5 forecasting questions on topic X. After this, a separate epistemic system will be run with a fixed number of resources R on each question. Choose questions such that the final results are maximally useful to end-users."
8. Forecasting
- Convert fetched data into a forecast.
- Can call the “Numeric Modeling” module, or be merged with it.
- There’s been previous work here that is promising.
- Fine-tuning and prompt engineering seems like it would go a long way.
- Current LLMs are known to be biased and often suffer from poor calibration, in part in order to become better liked by human users. We might well want specialized systems optimized for pure accuracy.
- This delta might cause problems. Perhaps it could lead to bad news stories (where a model used for forecasting gives a politically-incorrect answer), or perhaps it could be used by bad actors.
- This area should be thus treated with care. Perhaps some models are only available to certain researchers, or perhaps there should be large disclaimers provided for regular people attempting to use them directly.
- Forecasting can output high-level stories, probabilities, scorable functions, or other. There are many potential formats here.
- Example performance question:
“Using information I, and N LLM calls, make your best forecast on variable V. You may use the Numeric Modeling module when doing so, but this will eat into the LLM calls.”
9. Forecasting Resolution
- Most forecasting questions should be resolvable automatically. This can be done by either querying external data, or perhaps by asking a person/LLM for their opinion.
- This component is likely to often just either be, or defer to, other LEP modules.
- Question Creation and Optimization
- A strong LEP would make good judgment calls on what research or forecasting topics would be valuable to people, and initialize those.
- This could range from “once a user logs in, generates custom information for them to maximize their EV”, to, “write a list of standard forecasting questions that are collectively high-EV to a subpopulation.”
- Example performance question:
“Using an existing LEP L, and resources R, write a set of forecasting questions. Expect that forecasting resources F will be used on these questions, and you are trying to optimize utility function U.”
10. Presentation
- Many custom charts and graphics would likely be useful.
- Decisions need to be made about what final data should be shown to users and how.
- Automated decision-making: In some cases / eventually, LEPs would interact directly with decision systems. In theory, people don’t need to know almost anything - critical information could be used directly by in-world automations. For example, instead of an LEP doing research into the costs and benefits of vitamin D supplementation and showing you that research, a system instead just starts an order for you to get a regular set of supplements. This could come with optional research, but in many cases the research won’t be reviewed, if the system is trusted.
- In cases where LEPs are used behind APIs, the presentation layer could include answering questions about what the LEP could do, and suggesting specific uses for it.
- Presentation/wording should be optimized to be understandable to humans. There should be tests of the error rates of what was intended to be stated, and what humans understood. For example, many people have significant misunderstandings about basic statistics concepts, so probabilities might need to be handled with care.
- Example performance questions:
- “Using LEP output O, design a dashboard that user U will most prefer.”
- “Using LEP output O, design a set of complicated diagrams without detectable errors.” (judged by an LLM).
11. Distribution
- Full custom user interfaces could be created. This might range from a simple custom LLM chat, to a auto-generated set of dashboards, with the ability to delve deeper into any topic the user clicks on.
- LEPs could be accessed by any other chat LLM interface, as long as that interface can call external APIs. This could be generally useful for integrating into other AI tools and workflows, perhaps via other common LLM chat UIs.
- LEP information could be distributed like how human communication is communicated. Text messages, emails, Tweets, voice assistants, etc. Ideally the LEP could vaguely predict what information is useful to people and when, and then send them that information in those cases.
- Example: You get an email in the morning. It says, “Some news came out at 6am today that changed my recommendation of your financial assets. I recommend you change to a different mutual fund. I estimate that doing so will net you an average of $50/month.”
- Example performance questions:
- “Using LEP output O, design and implement the best way to get this information to agent A.” We can use an LLM that mimics human behaviors to evaluate.
- “Using LEP output O, design and implement the best way to get this information to agent A.” We can use an LLM that mimics human behaviors to evaluate.
12. Idea Generation / Optimization
- It will often be useful to generate ideas on the best ways to do something.
- For example, an LLM agent might want to make a suggestion to a user, before doing forecasting on said suggestion.
- Regular LLM performance might be fine on this, but fine-tuning or similar might help. It might be the case that there are certain specific domains where idea generation is very useful.
- “Idea Generation” is related to program optimization and other CS topics.
- Example performance questions:
- “Given a person with details D, generate a plan for that person to optimize their financial future.” (This will be then judged with an LEP / forecasting module)
- “Given a 2d grid game G, come up with 10 ordered ideas for the best strategy” (Can be judged empirically)
13. Task Orchestration
- We need systems that can actually trigger all the other components when needed. This process needs to be debuggable / introspectable. Ideally, these workflows would be highly understandable.
6. Benchmarks & Baselines
Claim 1: Accessing forecasting quality and efficiency with humans is very difficult and often poorly done.
- Scores like the Brier Score don’t take into account the difficulty of the question.
- It’s really hard to standardize question sets, audiences, and environments. Each time a human forecasting competition is done, it’s done with a separate population. Also, we typically don’t track the resources going into these systems - like the total time that people spend forecasting. Again, humans are mediocre for studying, in comparison to LLMs.
- We arguably don't yet have good statistical measures for credal resilience.
Claim 2: Portable LEPs could be useful for acting as priors or baselines, for other systems to perform against.
- We will want some baseline LEPs to score other LEPs against. These should act on the same data, at the same times.
- For example, one could take a new LEP, a well-known Baseline LEP, and a known or generated question set. Both systems would forecast those questions, and then the new LEP will be evaluated based on how much it outperforms the Baseline LEP. For example, with the average difference in brier score.
- In order to do this, the LEP must be equipped with the abilities to fetch data and to forecast. This is useful in order to compare the system to human forecasters (which do both), but it might be often better to divide the subcomponents and study/optimize them in isolation.
- Another use is to use Baseline LEP to initialize forecasting markets. Right now manifold questions begin at 50%. It could be better for them to instead begin at a number suggested by a simple LEP.
- Even if these LEPs aren't highly accurate, they could be useful for sanity checking claims outside of forecasting systems. For example, all publicly given estimates and statements could be run against LEPs. This can then highlight estimates that are particularly surprising. In financial / numeric models, all inputs and assumptions can be checked against LEPs to find ones that might be suspicious.
Claim 3: Standardized LEPs could be great judges to resolve subjective questions
- It’s well-known that it’s difficult to use partially-subjective questions in prediction markets. Much of the reason is because the only way to resolve these is with human expert judges, and these human judges have eccentricities that are hard to predict. AI systems might help here, because they can be far more standardized and testable than human systems.
- For example, say there’s a question, “Will project X generally be considered a failure in 2026?” This can be changed to, “Will project X be considered a failure, in an average of 20 runs, by a certain LEP, in 2026?” Then, predictors could provide this LEP fake hypothetical information on the future and see how this question would resolve with that information. There should be very little surprises from the eventual output of the LEP.
- Related, LEPs could be used to provide independent resolutions for every other forecasting question on Metaculus/Manifold/etc. There would be a certain expected error rate - if some resolution judge statistically exceeds this rate, that would be flagged for review.
Idea: It’s possible we could get very useful small LEPs that are easily packaged, relatively cheaply.
- Certain LEP components are much more useful to include for different kinds of testing than others. Some simple web search functionality and forecasting abilities could get us far for testing forecasting. Right now there are a bunch of startups and tools emerging to help with certain kinds of scaffolding that might be useful here. For example, you can currently bundle special data and prompts with a custom ChatGPT GPT. Right now this cannot be accessed via the API, but this is a potential thing that could exist in the future.
- One viable solution could be for someone to set up a very simple web service that can make internet queries and forecasts. Ideally, this would provide multiple immutable options, and this would be trusted to stay up, for future experimentation. It might only need to be accessible via an API.
- It might also be possible to have a simple LEP in a single Docker container or similar.
- Even very simple LEPs could act as much better baselines than what we use today.
Challenge: LLM-forecasting abilities will vary based on date.
- The quality of LLM predictions is likely to go down, the further away the forecasting question resolution is from the LLM training date. This is a major challenge to using them as a benchmark. For example, GPT4 might be decent at guessing the US GDP in 2025 if used in 2024 with search capabilities (i.e. web search). But if it were used in 2030, for the US GDP in 2031, with search capabilities but no other training updates, its performance would likely be worse. It's background/training data would be much less relevant.
- It's possible that there could simple numeric adjustments for this, for certain accuracy scores. For example, perhaps it's expected that the forecasting quality would decline according to a linear trend, in proportion between the time the LLM was trained, and when the forecasting question was asked.
- LLMs should rarely be used to “forecast” events that happened before their release. It’s likely that their training data would have leaks of the results, or of things close to these results.
- Related, LLMs will be better at forecasting in some domains in others. However, I don't think that this will be a major problem. We will learn about these boundaries over time, and I expect that they will be heavily correlated between different LLMs and LLM versions.
7. Caching vs. On-Demand Forecasting
Key question: How much would we expect AI forecasting systems to generate forecasts and visualizations on-demand for users, vs. save them before?
- On-demand work is more user-customizable and user-specific, but it’s harder.
- In the beginning, on-demand AI work will be used for the “last-mile” problem. A user asks a question - we first find the most similar forecasts, then use an LLM to use those to generate a personalized forecast. We’d also want great charts ready-to-go.
- This tracks a question about web content more generally. Eventually websites might be generated on-demand, to be personalized for users. But it will likely take some time to get there.
Simple heuristics:
- Save all generated forecasts, especially if these are expensive to produce. Reference them for future forecasting. This should be very useful to have the system cost-effectively scale to handle a large number of queries.
- Be careful of potential privacy issues, though.
- If the system can predict that (A) a bunch of questions will be asked on topic T in the future, and (B) some saved computations will be useful, then it will aim to produce such work ahead of time. This might be by fetching a lot of relevant data, updating the world model, or making other improvements.
- It’s probably not an efficient bet to make complex scorable functions with a lot of attention to parts of the parameter space that are unlikely to actually be called. Instead, do a rough version, then wait until they are called to bother improving them in these areas. Save the updated version, of course.
Further Research
I feel like there are clearly some larger investigative projects to do here. It's a vast and important topic, and at the same time there's a lot of fairly-obvious things (yet interesting and useful) we could say about it. This is mostly just putting a bunch of existing ideas in one place and providing some definitions and clarifications.
Some future things I'd like to explore (or have others explore!) include:
- Exploration of benchmarks and optimization of end-to-end LEPs
This document includes more about specific subcomponents of LEPs than the full thing itself. - Strategies for prioritizing philanthropic LEP work
I think this document helps list out a lot of potential LEP work, but it doesn't try to prioritize it. This would take some thinking. Maybe predictions can be used. - Cost-effectiveness estimation
I think these systems are promising, but I'm not sure how to best estimate the cost-effectiveness of them vs. other effective altruist interventions. It would be useful to have better models of benefits and risks, perhaps with new delineations. - Concrete project ideas for enthusiasts
I could imagine a lifehacker-like community forming around some of these principles. I think creative experimentation by enthusiasts could be useful, in part to help identify promising full-time talent. - Moving key claims and cruxes to forecasting platforms and LLMs
Fitting with the theme, it seems interesting to try to pull out key questions here and post them to forecasting platforms. I tried to isolate claims and cruxes, in part so that they could later be easily adapted into somewhat-concrete questions.
My guess is that a lot of people will bounce off this broad topic. I imagine that there's much better writing that could be done to formalize and popularize the key concepts that will be useful going forward. I'd encourage others to make stabs here.